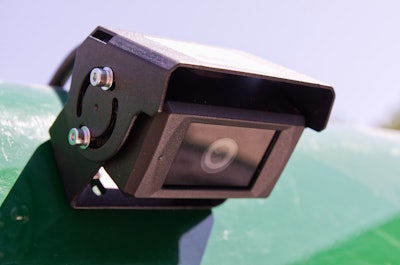
For years, artificial intelligence (AI) has been cited as the answer for a more advanced and streamlined experience that touches every aspect of how humans live and work. Now, in the age of “big data,” the application of AI is fast becoming something that many industries are using in abundance, including the improvement of road and worksite safety.
Five years ago, 360-degree bird’s-eye view cameras were the latest innovation. Now, this concept has been upgraded with AI technology that can recognize people and provide an active warning. Meanwhile, radar detection technology has moved from 24Ghz to 77Ghz, giving a more accurate, faster detection at a greater range. Moving even further still, the latest version can analyze the track of vulnerable road users and provide warnings only when there is a risk of a collision, therefore eliminating irrelevant warnings for objects and improving safety parameters.
However, there are still many challenges to using AI in vehicle safety devices. One of the keys to successful AI application is demystifying what this really means for end users. There are questions to AI’s learning and thinking capabilities and wariness that this could cause potential danger to humans on roads or worksites. In automotive technology, meticulously validated data sources are being applied through machine learning, primarily used for image detection. The AI for vehicle electronics, which is “pre-trained’ before install, is programmed to operate in a specific way. For example, AI is programmed to detect particular situations or vulnerable road users, such as pedestrians or cyclists, and therefore cannot begin to develop a life of its own and make decisions for itself — a common misconception. To overcome misconceptions such as this, consider the following.
1. How can AI improve road and worksite safety?
AI has enormous potential to improve safety on roads and worksites, but it is dependent on pre-learned scenarios and precision. It draws on its data sources and makes a detection decision based on this information, so any AI used in automotive technology must use validated data sources. With this, there are secure, pre-trained and programmable actions defined in a specific way. For example, a camera will have been programmed to respond in a certain way if a cyclist veers in front of a vehicle. This pre-programming means it will make the same decision every time, without exception, because it is a predetermined pattern. This eliminates detection decision-making, which could potentially lead to catastrophic outcomes. The machine is not defined by emotion, but by a pre-defined pattern, so it’s more decisive. When it needs updating, the current algorithm can be changed for a new and more refined version.
2. Are there concerns about how much AI is taking over?
In the vehicle safety industry, AI should be able to avert for potential collisions across driver assistance systems, self-driving vehicles, intelligent traffic management systems, etc., all based on data-driven decision-making. The potential for safer roads is huge. Currently, AI is being used as a supportive system and, provided the checks are in place, it is a reliable method of enhancing safety, which is a positive step forward. In this industry, there would be much more concern if products came to market that had not been tested exhaustively.
3. Can AI fail or get things wrong?
In the vehicle safety market, this is arguably one of the biggest concerns. We’ve all read about chatbots giving misleading, legally questionable, and even potentially dangerous advice to people because the bot has collated its response from random or non-validated sources. AI training is similar to human learning — the information on which it bases its response has to be credible and true. If it isn’t, then issues can arise. It is essential that AI be tested in the real world because there will always be new situations not yet envisaged.
One example of this is a site worker in a high-visibility vest. If the AI is not trained effectively, the glare from the vest can create a halo effect and it will be impossible to guarantee detection. There are also cases in which sunlight wipes out an image, bringing a vision-based AI system to its limit. These issues are being improved alongside the advancements in camera technologies, as well as fusion with other sensor technologies, e.g., radar. Overall, the better the information provided to an AI system, the more you can get out of it.
4. What are the benefits of AI road safety systems?
Traditional detection systems measure physical dimensions, but don’t classify what something actually is. They measure it and draw a conclusion as a result. With AI, there is an ability to classify an object, such as a cyclist, pedestrian, moving car or safety barrier. Humans may respond randomly to sudden incidents; however, a machine will always come up with a predefined reaction that is often safer — but it depends on the intensity of its training.
And if something goes wrong, there will always be the issue of liability. Is an incident the responsibility of the driver, the manufacturer, the AI training material, a combination of the three — or none of these? In my opinion, at the moment, the best compromise for an application is a combination of AI and both image data and traditional radar detection technology, but this may change with AI advancements and, furthermore, with the addition of a third sensor technology.
In investigating the use of AI and whether it can enhance road and workplace safety further, a camera that can detect pedestrians using AI with state-of-the-art machine learning was first up, using AI to accurately evaluate received images in cameras fitted to vehicles and calculate when nearby people or objects became critical. Its success inspired expanded research into AI safety devices.
The latest cameras have larger detection areas, recognize humans using AI, and warn the driver of a vehicle visibly and/or audibly about a possible hazard. The system has a high-definition (HD) or higher-resolution vison sensor with all processing power embedded into the camera assembly, and the detection range stretches from the front of the vehicle to several meters out. The camera’s computer uses machine learning to improve detection rates continually via a thoroughly tested state-of-the-art algorithm.
Engineers have spent long hours to ensure these systems can cope with any situation in the shortest possible reaction time. And it’s not just a question of identifying a human; every practicable scenario has been tested. The human in question could be 6 feet, 4 inches tall or a child at 3 feet fall. They could be standing, running, wearing a large hat, operating a forklift, pushing a wheelbarrow, sitting down, riding a bike — initial adjustments were even included to allow for a human lying down. Another scenario, training AI to recognize speed differentials and track objects, can identify the position of a cyclist versus another vehicle in relation to movement.
5. Are more AI advancements coming?
Over the years, technology has progressed from the early days of vehicles providing warnings, such as low-fuel indicator lights, to the active assistance of today’s detection cameras. Cameras can currently help drivers stay in the correct lane on a highway, detect vulnerable road users, and warn us of activity in blind spots. Fully automated cars are already becoming a reality — this market will be one to watch. Further advancements in AI are inevitable, but if they continue to prevent death and injury on roads and worksites, then we must do everything we can to channel them securely and responsibly. It’s important to remain aware of the operator workload that these new systems may present.
It’s also crucial for technology partners to work together to package multiple sources of information, both active detection technologies alongside passive technologies, into one display. The active elements will draw the attention of the operator, providing visible or audible warnings, while the passive elements provide an immediate overview of the situation.
Another area now gaining traction is sensor fusion technology. Teams working with OEMs on combining different technologies, such as cameras and radar, can provide a more holistic coverage that any one system can achieve alone.
Mike Hardman is the engineering and technical services manager at Brigade Electronics Inc.