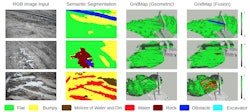
Researchers from Baidu Research Robotics and Auto-Driving Lab (RAL) and the University of Maryland, College Park (UMD) have developed a real-time mapping approach for autonomous navigation of excavators on complex terrains named Terrain Traversability Mapping (TTM). Using TTM, an autonomous excavator can navigate through unstructured outdoor environments consisting of deep pits, steep hills, rock piles, and other complex terrain features. This is the first complex terrain processing approach developed for heavy-duty excavation machines.
The research team exhibited the technology at MINExpo INTERNATIONAL 2021. MINExpo 2021 boothBaidu Research Robotics and Auto-Driving Lab
The development of robotic systems such as autonomous excavators in the construction and mining industries is a growing trend as these companies worldwide are encountering labor shortages in the face of increasing demand for skilled heavy machinery operators. The global market size for excavators was $44.12 billion in 2018 and is expected to grow to $63.14 billion by 2026.
Excavators are widely operated in unstructured and dangerous environments that have unpredictable and potentially hazardous conditions. The operations of excavators consist of digging and dumping, which raise the risk of landfalls or cave-ins. These complex environments lack lane markings, and obstacles tend to be non-uniform and random, making them less navigation-friendly. To ensure the safety of operators in this environment, it is crucial for autonomous excavators to be able to identify different terrain features and predict safe regions for navigation.
To enable autonomous excavators to handle complex terrains, the researchers developed an efficient learning-based geometric method to extract terrain features from RGB images and 3D point clouds and incorporate them into a global map for planning and navigation. The method uses physical characteristics of the excavator, including maximum climbing degree and machine specifications, to determine the traversable area, adapt to changing environments, and update the terrain information in real-time. In addition, these researchers have prepared a novel autonomous excavator terrain dataset, which consists of RGB images and LiDAR point clouds from construction sites with seven different categories based on navigability. This integrates the mapping approach with planning and control modules to continuously improve the autonomous excavator navigation system. Experiments showed that while using TTM, an excavator can navigate through unstructured environments with a much higher success rate compared to existing planning schemes.
The new TTM technique comes on the heels of another innovation Baidu RAL and UMD co-developed this June -- an autonomous excavator system (AES) that can perform material loading tasks for 24 hours without any human intervention, while offering performance nearly equivalent to that of an experienced human operator. The researchers described their methodology in a research paper published on June 30, 2021, in Science Robotics.
Baidu Research Robotics and Auto-Driving Lab